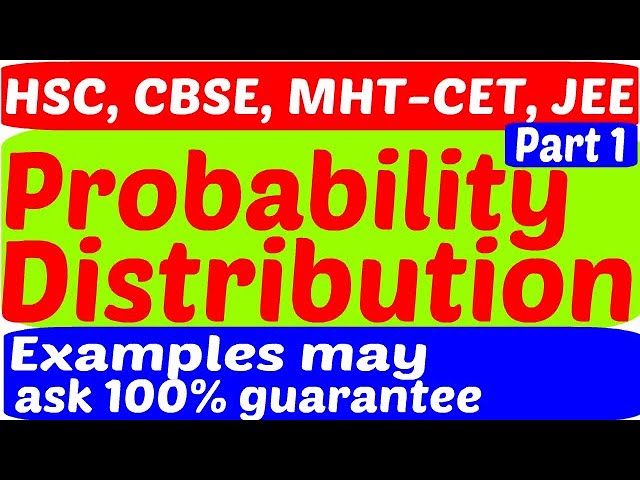
In this video we are going to see some important examples on probability distribution.
The topic carries 05 marks with option for HSC Board and 6 marks with option for CBSE Board and study of this topic definitely helpful for the preparation of MHT CET and JEE Main Exam.
This topic is very simple like Logic, matrices and LPP no trigonometry, no limit, no derivative, no hard integration. For this chapter you require a basic knowledge of probability from 11th std. and some basic knowledge of definite integrals.
So let’s begin. First we shall see what is meant by random variable, probability distribution, probability mass function (p. m. f.), and cumulative distribution function (c. d. f.) with some examples.
Consider an example of tossing 3 coins simultaneously. The Sample space in this case is
S= {HHH, HHT, HTH, THH, HTT, THT, TTH, TTT}
Let X denote number of heads occurs
Hence X takes the value 0,1,2,3.
P [X= 0] = P (0) = 1/8
P [X= 1] = P (1) = 3/8
P [X= 2] = P (2) = 3/8
P [X= 3] = P (3) = 1/8
The X is called a Random variable and P (0), P (1) ... are called probabilities and the function P is called probability mass function (p. m. f.) of X.
Hence the required probability distribution is
X=x 0 1 2 3
P[ X=x] 1/8 3/8 3/8 1/8
From this table we observed that p. m. f. of random variable satisfy
a) 0 less than or =p less than or = 1 and
b) sum of all the probabilities = 1
i.e. P (X=0) +P (X=1) +P (X=2) +P (X=3) =1
In above example the random variable X is discrete variable. Discrete variable means the variable that takes values as non-negative integers.
In my next video we are going to discuss probability distribution of continuous variable.
Ex. 1. Verify whether each of the following functions can be regarded as p. m. f. for the given value of X
(1)
X=x -2 -1 1 2
P[ X=x] 0.5 -0.1 0.6 0
(2) P(x)={█((x-1)/3,&x=1,2,3@0, Otherwise.)┤
Solution:
p. m. f. of random variable satisfies a) 0 less than or =p less than or = 1
and b) sum of all the probabilities = 1.
(1) Here P (X=-1) = -0.1 less than 0.
Hence, P(X=x) cannot be regarded as p. m. f. of random variable X.
(2) Here
P [X= 1] = P (1) = 0
P [X= 2] = P (2) = 1/3
P [X= 3] = P (3) = 2/3
Hence (a) 0 less than or =p less than or = 1
and b) sum of all the probabilities =
P (1) +P (2) +P (3) =1.
Hence, P(X=x) can be regarded as p. m. f. of random variable X.
Ex.2. Two cards are drawn from a pack of 52 cards. If X= number of red cards drawn, find the p. m. f. of X.
Solution: Two cards are drawn from a pack of 52 cards in 52C_2 ways.
Hence n(S) =52C_2
Since X = number of red cards
Hence X takes the values 0,1,2
P (X= 0) =P (0) = P (no red cards) = P (both black cards) =〖26C〗_2/〖52C〗_2 = 26x25/(52x51 ) = 25/102
P (X= 1) =P (1) = P (1 red card and 1 black card)
= (〖26C〗_1 x〖26C〗_1)/〖52C〗_2 = (26x26/1x1)/(52x51/2x1) = 26/51
P (X= 2) =P (2) = P (both red cards)
=〖26C〗_2/〖52C〗_2 = 26x25/(52x51 ) = 25/102
Hence p. m. f. of X is given by
P (0) =25/102 , P (1) =26/51, P (2) = 25/102
Ex. 3. Determine k such that the following function is a p. m. f.
P(x)={█(k(4¦x),&x=0,1,2,3,4;k greater than 0@0,&Otherwise.)┤
Solution:
The symbol (4¦x) = 4Cx and we use the formula n Cr= n C(n-r)
Hence P(X=0) = kx4C0 =k (1) =k
P(X=1) = kx4C1 =k x (4) =4k
P(X=2) = kx4C2 =k x(4x3/2x1) =6k
P(X=3) = kx4C3 =k x (4) =4k using 4C3= 4C1
P(X=4) = kx4C4 =k (1) =k using 4C4= 4C0
Since P(X=x) is a p. m. f., Sum of all P (X=0 to 4) = 1
. P (0) +P (1) +P (2) +P (3) +P (4) =1
Hence k + 4k + 6k+ 4k+ k = 1
Hence 16k = 1
Hence k= 1/16.
That’s all about this video. In my next video on probability distribution we shall see some more examples.
Thank you for watching my video.
0 Comments